14
Víctor Bucarey ● Profesor Asistente
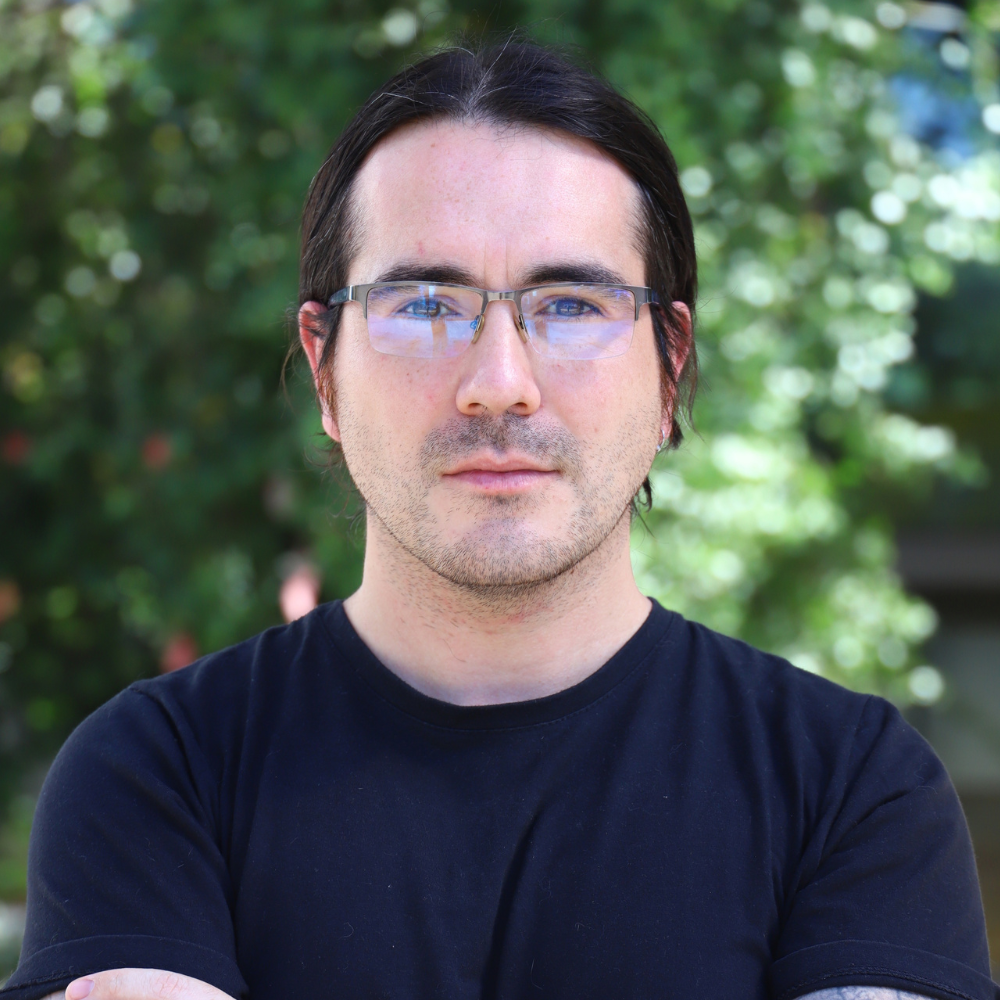
Grado Académico
Doctor en Sistemas de Ingenieria, Universidad de Chile
Título(s) Profesional
Ingeniero Civil Industrial
Descripción
Víctor Bucarey es Ingeniero Civil Industrial, con un doctorado en Sistemas de Ingeniería de la Universidad de Chile. Actualmente es profesor Asistente en la Universidad de O’Higgins. Sus intereses de investigación se centran en la toma de decisiones basadas en datos, teoría computacional de Juegos y la optimización a gran escala. Actualmente, se encuentra trabajando en aprendizaje para problemas difíciles de optimización combinatorial y algoritmos eficientes para resolver problemas de predicción + optimización. Además, durante su carrera ha colaborado en variados proyectos de divulgación científica.
Víctor integra el área de Investigación de Operaciones del Instituto de Ciencias de la Ingeniería.
6
- REVISTA Computers and Industrial Engineering
- 2024
Probability estimation and structured output prediction for learning preferences in last mile delivery
• Rocsildes Canoy • Víctor Bucarey • Jayanta Mandi • Maxime Mulamba • Yves Molenbruch
- REVISTA Omega
- 2024
Playing Stackelberg security games in perfect formulations
• Pamela Bustamante • Víctor Bucarey • Martine Labbé • Vladimir Marianov • Fernando Ordoñez
- REVISTA Constraints
- 2023
Learn and Route: Learning implicit preferences for vehicle routing
• Rocsildes Canoy • Víctor Bucarey • Jayanta Mandi • Tias Guns •
- REVISTA IEEE Transactions on Automatic Control
- 2022
Stationary Strong Stackelberg Equilibrium in Discounted Stochastic Games
• Víctor Bucarey • Eugenio Della Vecchia • Alain Jean Marie • Fernando Ordoñez •
- REVISTA Proceedings of Machine Learning Research
- 2022
Decision-Focused Learning: Through the Lens of Learning to Rank
• Jayanta Mandi • Víctor Bucarey • Maxime Mulamba • Tias Guns •
- REVISTA 2022 24th European Conference on Power Electronics and Applications (EPE'22 ECCE Europe)
- 2022
A Non-cooperative Game-theoretic Distributed Control Approach for Power Quality Compensators
• Claudio Burgos Mellado • Víctor Bucarey • Helmo K. Morales-Paredes • Diego Alejandro Muñoz Carpintero •
- REVISTA Computers & Operations Research
- 2021
Models and algorithms for the product pricing with single-minded customers requesting bundles
• Víctor Bucarey • Sourour Elloumi • Martine Labbé • Fränk Plein •
- REVISTA European Journal of Operational Research
- 2021
Coordinating resources in Stackelberg Security Games
• Víctor Bucarey • Carlos Casorrán • Martine Labbé • Fernando Ordoñez • Oscar Figueroa
- REVISTA Omega
- 2021
An exact dynamic programming approach to segmented isotonic regression
• Víctor Bucarey • Martine Labbé • Juan M. Morales • Salvador Pineda •
- REVISTA International Game Theory Review
- 2021
The Stackelberg Games of Water Extraction with Myopic Agents
• Mabel Tidball • Alain Jean Marie • Víctor Bucarey •
- REVISTA Proceedings of the Thirtieth International Joint Conference on Artificial Intelligence
- 2021
Contrastive Losses and Solution Caching for Predict-and-Optimize
• Maxime Mulamba • Jayanta Mandi • Michelangelo Diligenti • Michele Lombardi • Víctor Bucarey
- REVISTA Lecture Notes in Computer Science
- 2019
Discussion of Fairness and Implementability in Stackelberg Security Games
• Víctor Bucarey • Martine Labbé •
- REVISTA Lecture Notes in Computer Science
- 2017
Building Real Stackelberg Security Games for Border Patrols
• Víctor Bucarey • Carlos Casorrán • Oscar Figueroa • Karla Rosas • Hugo Navarrete
- REVISTA Applications of Location Analysis
- 2015
Shape and Balance in Police Districting
• Víctor Bucarey • Fernando Ordoñez • Enrique Bassaletti •
- REVISTA 24th European Conference on Power Electronics and Applications (EPE'22 ECCE Europe)
A Non-cooperative Game-theoretic Distributed Control Approach for Power Quality Compensators
• Claudio Burgos Mellado • Víctor Bucarey •
- FONDEQUIP EQM230041
- Enero 2023
- - Enero 2026
Proyecto Adjudicado
Consiste en diversos módulos de baja potencia que pueden configurarse e
interconectarse para implementar variadas topologías emergentes de
sistemas eléctricos y topologías de conversión como: microrredes, enlaces
de alto voltaje en corriente continua (HVDC), convertidores modulares
multinivel (MMC), sistemas de baterías (BESS), cargadores rápidos, entre
otros. Cada módulo de potencia posee una unidad de control propia
coordinada por una unidad central, lo que permite implementar esquemas
de control distribuido. Además, la plataforma contempla una etapa de
amplificación de potencia trifásica, que permite generar físicamente los
voltajes y corrientes de un punto común de acoplamiento con una red
eléctrica emulada en tiempo-real. Esto permite estudiar la interacción de la
red emulada con los sistemas eléctricos y las topologías de conversión
emergentes descritas anteriormente. Por consiguiente, esta plataforma
agiliza el prototipado, tanto en hardware de potencia como de control,
permitiendo la validación experimental de estrategias de control
distribuido que, a diferencia del control centralizado (tradicionalmente
utilizado en la academia e industria), presenta ventajas que son de utilidad
para mejorar la resiliencia de los sistemas eléctricos, como son: mejor
confiabilidad, flexibilidad, escalabilidad, operación plug-and-play y
tolerancia a fallas de un solo punto.
- Enero 2023
Proyecto Adjudicado
La crisis hídrica provocada por el calentamiento global es uno de los problemas más importantes que afectan a regiones agrícolas como la sexta Región de OHiggins. Este proyecto de vinculación internacional tiene como objetivo principal investigar distintos mecanismos para la asignación de recursos hídricos e épocas de escasez. En particular, se analizará el caso en que se tienen multiples usos de agua: distintos tipos de industrias (cupríferas y agrícola por ejemplo) y uso urbano. Este proyecto de vinculación internacional es liderado por el equipo nacional conformado por investigadores de la Universidad de O'Higgins y Universidad de Chile. Este proyecto incluye visitas del equipo nacional a los centros INRIA Sophia Antipolis, y INRAE en Montpellier, así como la organización de un workshop en la Universidad de OHiggins.
- Enero 2023
- - Enero 2024
Proyecto En Ejecución
La crisis hídrica provocada por el calentamiento global es uno de los problemas más importantes que afectan a regiones agrícolas como la sexta Región de OHiggins. Este proyecto de vinculación internacional tiene como objetivo principal investigar distintos mecanismos para la asignación de recursos hídricos e épocas de escasez. En particular, se analizará el caso en que se tienen multiples usos de agua: distintos tipos de industrias (cupríferas y agrícola por ejemplo) y uso urbano. Este proyecto de vinculación internacional es liderado por el equipo nacional conformado por investigadores de la Universidad de O'Higgins y Universidad de Chile. Este proyecto incluye visitas del equipo nacional a los centros INRIA Sophia Antipolis, y INRAE en Montpellier, así como la organización de un workshop en la Universidad de OHiggins.
- Enero 2023
- - Enero 2024
Proyecto En Ejecución
Development of algorithms for bilevel optimization problems with applications in security, electricity and logistics.
- Enero 2023
- - Enero 2024
Proyecto Adjudicado
Decision-making processes involving uncertainty in the input parameters are commonplace, and the operational research community has investigated different methods to tackle this issue since the 60s. The current widespread availability of data and information presents a great opportunity to enhance the decision-making process, but at the same time, it presents a great challenge to develop new models and algorithms that capture this information. Stochastic programming, robust optimization, risk-averse optimization and distributionally robust optimization are different ways to deal with uncertainty. The input parameters are either modeled as random variables with a probability distribution that can be estimated from data; or as an uncertainty set which is often also decuded from data. A different approach is decision-focused learning or (a.k.a. smart predict-then-optimize) in which input parameters are learnt by a machine learning model which is trained considering the impact on the sub-opmality decisions made by not knowing perfectly the uncertain parameters. After training with this methodology, uncertain parameters are treated as deterministic parameters for the optimization problem. The main objective of this project is to provide a general framework for data-driven decision-making for different settings in transportation and logistics and to provide large-scale optimization models and algorithms to tackle uncertainty when data is available for these settings. We aim to compare and understand when each methodology to deal with uncertainty is the most adequate. Specifically, we aim to study problems in two-tier city logistics; large scale shortest path problems with real data motivated by firetruck dispatchment; multi-layer districting problems with balance constraint and uncertain demand; and the allocation security resources in a transportation network where the behavior of opportunistic passengers and the deployment of security agents are correlated.
- Marzo 2022
Proyecto En Ejecución
Investigador/a Responsable
Numerous real-life decision-making processes involve solving a task with uncertain input that can be estimated from historic data. There is a growing interest in decision-focused learning methods (a.k.a. smart predict-then-optimize) whose goal is to find models that fit the data while considering how the predicted input will perform in a particular task. For example, the task can be a shortest path problem that uses predictions on travel times in the objective function. Fitting the data and ignoring the task may lead to sub-optimal decisions. Sometimes, uncertainty is involved in the constraints of the model. In this case, ignoring the task would lead to infeasible decisions. The goal of this project is to develop efficient exact algorithms and new applications to train a Machine Learning models that perform well in one or several tasks using mathematical programming (MP) tools.
In this context, the typical measure of a predictor is the regret: the excess cost incurred when making a suboptimal decision due to an imprecise predictor. This problem is bilevel in nature: the top-level decision consists in determining a predictor that minimizes a regret while considering that the predictions will affect a task, e.g. an optimization problem in a lower level. This structure is typically non-convex and non-continuous, making the problem difficult to solve for realistic instances. However, several recent advances in bilevel-tailored approaches exploit this structure and can solve large scale problems.
There are two main ways of estimating task-oriented predictors: 1) stochastic gradient-based methods; and 2) MP reformulations of the problem. Stochastic gradient-based methods replace the non-differentiable regret for some differentiable surrogate loss function approximating the real loss. Due to advances in neural network implementations and stochastic gradient-descent this approach is the most applied among researchers and practitioners in the Machine Learning community.
MP for data science has attracted the attention of researchers and practitioners in different areas as mathematics, operations research, computer science during the last years. It provides some degree of flexibility, being able to model desirable considerations for predictions models. For instance, MP has been successfully used to train sparse models yielding improved explainability and/or fairness. Moreover, MP models are in many cases solvable by any off-the-shelf solver. However, for the decision-focused learning problem there are still many gaps using MP formulations. To the best of our knowledge, MP formulations have been used only for surrogate loss functions.
Behind the low usage of MP tools is the scalability. A typical ML setting involves data sets involving thousands of observations. In consequence, the training task becomes more difficult. To tackle this issue, decomposition methods such as cut and column generation can help to solve problems at scale. We aim to provide efficient exact methods that can return either optimal solutions, or optimality guarantees for large scale instances.
During the last years, decision-focused learning has been widely applied to combinatorial optimization problems. However, this approach can also be used in many other applications such as Markov decision processes (MDPs) or game theory. In the first case, we can take algorithmic advantage of well-known existing algorithms for MDPs such as value iteration or policy iteration, or approximation techniques such as Q-learning. The game-theoretic setting is more challenging: depending on the notion of equilibrium the loss function would change. For instance, the definition of regret can be applied straightforwardly to Stackelberg equilibria (or leader-follower equilibria), a concept widely applied to energy markets, security and transportation. In the case of Nash equilibrium, the definition of regret is not direct anymore. We hope to develop models that adapt the decision-focused learning paradigm to this broader context.
The study of this topic requires the use and development of MP of tools along with their algorithmic analysis. During the project, we expect to develop efficient of algorithms that can be used by decision making, as well as contribute in the understanding of the theoretical aspects of decision-focused learning.
- Abril 2018
- - Noviembre 2020
Proyecto Ejecutado