9
Alexander Baumgartner ● Profesor Asistente
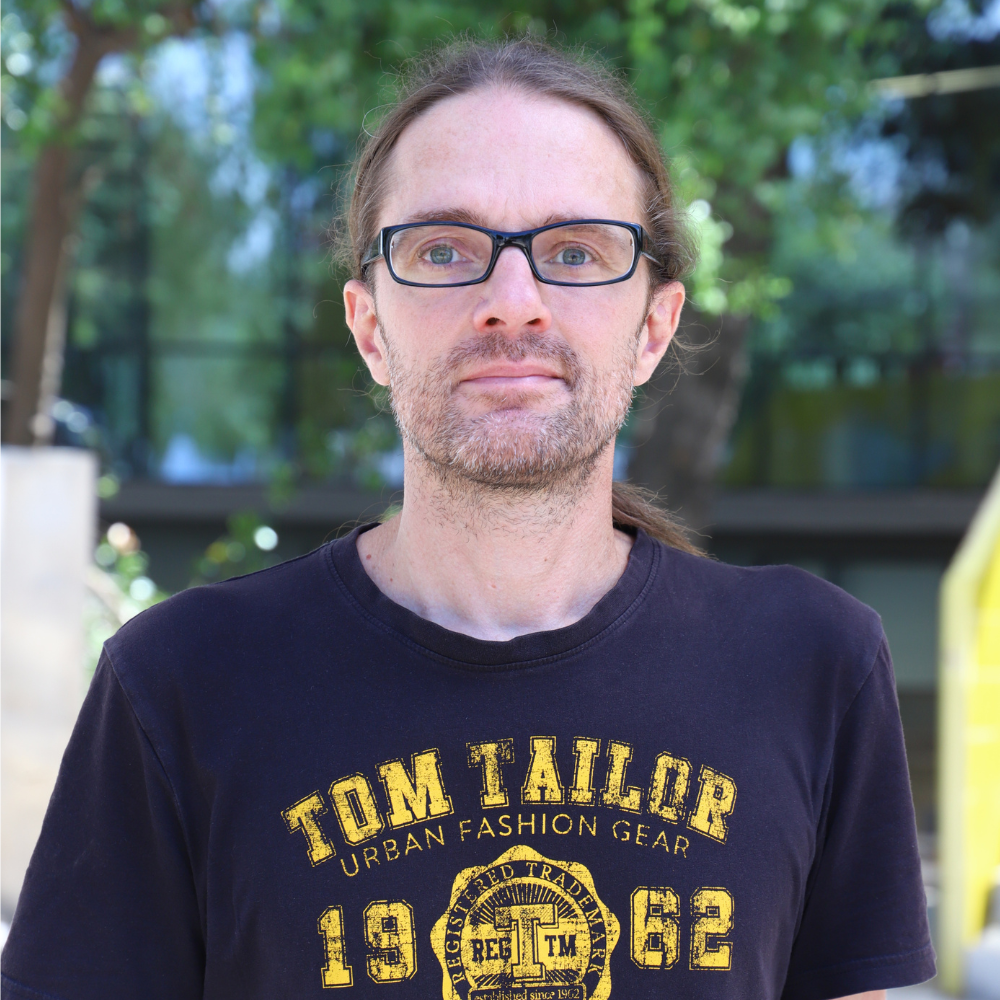
Grado Académico
Doktor der technischen Wissenschaften (Dr.techn.) Johannes Kepler Universität Linz, Austria
Título(s) Profesional
Diplom-Ingenieur (DI), Paris-Lodron University of Salzburg, Austria
Descripción
Doktor der technischen Wissenschaften (Dr.techn.) Johannes Kepler Universität Linz, Austria
2
- REVISTA LTCS-Report
- 2024
Towards a Well-Founded ≤ Relation for Permissive Nominal Terms
• Alexander Baumgartner
- REVISTA Proceedings of The 34th International Workshop on Unification
- 2020
A,C and AC Nominal Anti-Unification
• Alexander Baumgartner • Daniele Nantes-Sobrinho
- REVISTA Proceedings of the ACM Symposium on Principles of Database Systems
- 2019
Regularizing Conjunctive Features for Classification
• Pablo Barceló • Alexander Baumgartner • Victor Dalmau • Benny Kimelfeld
- REVISTA LIPIcs
- 2018
Term-Graph Anti-Unification
• Alexander Baumgartner • Temur Kutsia • Jordi Levy • Mateu Villaret
- REVISTA Journal of Automated Reasoning
- 2017
Higher-Order Pattern Anti-Unification in Linear Time
• Alexander Baumgartner • Temur Kutsia • Jordi Levy • Mateu Villaret
- REVISTA Information and Computation
- 2017
Unranked second-order anti-unification
• Alexander Baumgartner • Temur Kutsia
- REVISTA LIPIcs
- 2015
Nominal Anti-Unification
• Alexander Baumgartner • Temur Kutsia • Jordi Levy • Mateu Villaret
- REVISTA Lecture Notes in Computer Science
- 2014
A Library of Anti-unification Algorithms
• Alexander Baumgartner • Temur Kutsia
- REVISTA LIPIcs
- 2013
A Variant of Higher-Order Anti-Unification
• Alexander Baumgartner • Temur Kutsia • Jordi Levy • Mateu Villaret
- #635276
- Noviembre 2019 - Septiembre 2024
En EjecuciónAgencia Nacional de Investigación y Desarrollo - ANID
Supervised Learning Techniques for Relational Databases
In general, machine learning aims to learn a model from the input data in order to make reliable and repeatable decisions. The learning of a model is either done automatically or semiautomatically. While deep learning can be used to automatically learn a model from arbitrary raw data, the number of successful application domains is still very restricted.
This proposal is concerned with supervised learning - a machine-learning technique that aims at learning a model from input-output examples. A crucial task in supervised learning is the engineering of the features. Features are used to extract the relevant information from the raw data in order to learn a classifier that is based on the extracted data. A classifier is a function that partitions the input data into different categories. Feature engineering is a time-consuming process that includes a lot of trial and error, and stepwise addition or deletion of features. We aim at automating that process and learn a classifier based on some automatically generated features.
Co-Investigador/a
- #635276
- Noviembre 2016 - Octubre 2018
EjecutadoAgencia Nacional de Investigación y Desarrollo - ANID
Approximation of Conjunctive Query Evaluation
Problems that cannot be solved by classical computers in reasonable time due to their high computational cost arise in many research areas. In general, the evaluation of conjunctive queries over relational databases belongs to those problems. Conjunctive queries form the core of the Structured Query Language (SQL) which became a de facto standard for querying and maintaining relational databases. This work is about developing new approximation techniques for conjunctive queries which cannot be evaluated in reasonable time. Our new approximation techniques should lead to significant improvements for data aided decision making, e.g., for early warning system which are based on the analysis of big data or to make business-critical decisions by analyzing big data. In the last decades, a very good understanding of the classes of conjunctive queries which can be evaluated in reasonable time has been gained and it has been proven that an under-approximation of a query always exists within each of those classes. However this approach is rather strict and some of the under-approximations can be rather uninformative, i.e., the under-approximation might return the empty result set while the original query would not. over-approximations might be helpful when this happens, as they return all answers to a query. One of our goals is to study the foundational aspects of over-approximations, including the existence problem and the problem of computing an approximation. Unfortunately, over-approximations do not always exist (within a class of queries which can be evaluated in reasonable time), and it is not even known to be decidable whether a conjunctive query admits an over-approximation. Therefore, another goal of the proposed work is the development of more liberal approximation techniques that yield some kind of quantitative guarantees. This means that they should guarantee that the result of the approximation is not too far from the result of the original query over a set of databases of interest. Therefore we need to define a measure of disagreement between queries and/or results. For conjunctive query evaluation, such measures do not exist up until now. Based on that measure, we study approximations whose disagreement with the result of the query they approximate is below a certain threshold. Furthermore, we investigate how the underlying data of a database can help us to find better approximations.
It has been shown that there are close relations between the approximation of conjunctive queries over relational databases and some classes of Semantic Web queries over semi-structured data. We also study possible connections between our approximation techniques and approximating Semantic Web queries.
Co-Investigador/a
Mail de contacto